09 May 2025
Rethinking Quality Assurance Using AI and Computer Vision with Top Data Science
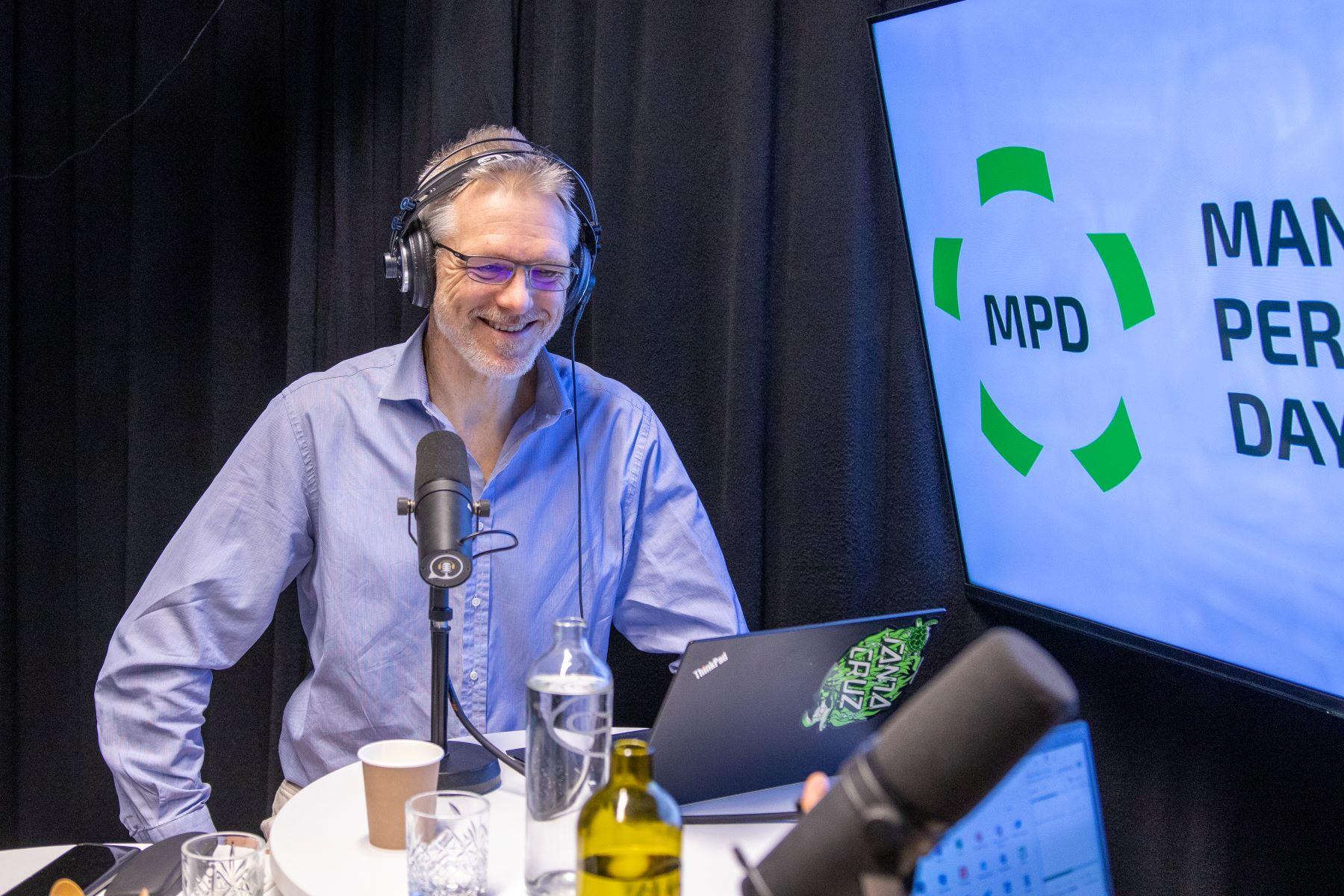
In manufacturing, quality assurance is both critical and costly, often reliant on manual inspection, inconsistent standards, and difficult-to-scale processes. But what if you could rethink the entire approach with AI and computer vision without needing large historical datasets?
In this episode of the MPD Q, A & I podcast, Kai Lehtinen, CEO of Top Data Science, breaks down how flexible, AI-based visual inspection can become a powerful tool to modernize quality assurance. His message is clear: you don’t need to wait for perfect conditions to start. In fact, data can be collected as part of implementation, removing one of the biggest perceived roadblocks.
“In most of our computer vision implementations, we collect data while we implement the solution. So you don’t need to have any preliminary data,” says Lehtinen.
Focus on the Business Problem, Not the Buzzwords
At the heart of Lehtinen’s approach is a simple but essential principle: organizations should begin their AI journey by clearly identifying the most meaningful business problem they want to solve through automation.
Too often, companies focus on AI as a technical trend rather than a tool to solve specific challenges. Lehtinen encourages a value-first mindset: start with the business pain point, define the desired end game, and only then explore the technology that best fits the goal.
“There will be tools and ways to achieve what you want to achieve,” says Lehtinen.
From Pilots to Scalable Impact
The discussion also covers how to scale from pilots to production. For Top Data Science, collaboration and communication are key. Lehtinen emphasizes co-designing solutions with end-users to make the shift smooth, understandable, and effective.
And while some believe you must already have large volumes of data to even consider AI, Lehtinen challenges that assumption directly: “Instead of thinking ‘can we do something because we don’t have data,’ you should be thinking ‘what do we want to achieve?’ It might be that you don’t need data.”
Measuring What Matters
In manufacturing, impact must be measurable. Lehtinen recommends tracking practical KPIs. These are the metrics that matter to the shop floor and the boardroom alike.
“Measure the time savings, raw material savings, waste reduced. Those are the best ways to track benefits,” he says.
Despite the growing complexity of AI, Lehtinen believes the barriers to entry are lower than many expect. It’s more about focus, not feasibility.
“In fact, it's quite easy to find use cases that complement what you already have.”
The MPD Q, A & I podcast series, part of the Manufacturing Performance Days, dives deep into the role of AI in creating new digital business models, advancing technologies, and leading sustainable transformation. Through candid conversations with leading professionals at the forefront of AI transformation, the series offers listeners first-hand insights into the future of AI-powered manufacturing.
Find the full episode on
Spotify
https://open.spotify.com/episode/0wSceYDrmCQ3ffo87sWWJ0?si=56d98424bedf4b19
Apple Podcasts